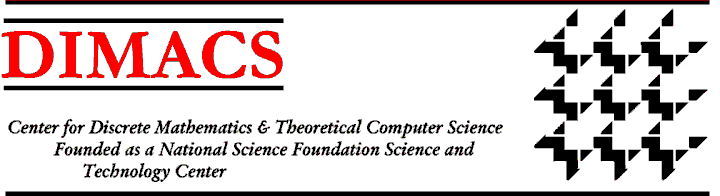
Differentially Private Modeling of Human Mobility at
Metropolitan Scales
[November, 2013] Former Rutgers graduate student Darakhshan Mir
(pictured)
and
her advisor DIMACS Director Rebecca Wright collaborated with Ramón
Cáceres (AT&T-Research), Sibren Isaacman (Loyola University
Maryland), and Margaret Martonosi (Princeton) to apply differential
privacy to human mobility modeling in metropolitan areas. Models
that can faithfully mimic human mobility have broad applicability in
public planning, ecology, epidemiology, and other fields. The
research of Mir and her collaborators adapts a previously-existing
approach for metropolitan mobility modeling using data from cellular
phone networks to add privacy guarantees. The goal of the new work
is to realistically model how large populations move within a
metropolitan area while rigorously safeguarding the privacy of
individuals whose data are used.
The previous approach, called WHERE, takes as input spatial and
temporal probability distributions drawn from empirical data, such
as Call Detail Records (CDRs), and produces synthetic CDRs for a
synthetic population as output. The synthetic output captures
distinct mobility patterns that arise because of differences in
geographic distributions of homes and jobs, transportation
infrastructures, and other factors. Its accuracy has been validated
against billions of location samples for hundreds of thousands of
cell phones in the New York and Los Angeles metropolitan areas.
Although WHERE intuitively affords a certain level of privacy
because it uses aggregated distributions of sampled and
straightforwardly anonymized data, a more rigorous assurance of
privacy would further advance safe and widespread use of such
models.
The work of Mir and her collaborators offers
this type of assurance with a “differentially private” variant of
WHERE, called DP-WHERE. It provides provable privacy guarantees by
adding a controlled amount of noise to the set of empirical
probability distributions that WHERE uses (for example distributions
of home and work locations). DP-WHERE then proceeds identically to
WHERE by systematically sampling these distributions to generate
synthetic CDRs containing synthetic locations and associated times.
The gray areas in the flowchart figure
show the places in which DP-WHERE differs from WHERE. Experiments
confirm that the accuracy of DP-WHERE remains close to that of WHERE
and of real CDRs.
Differential privacy makes privacy a mathematical requirement on the
results of interactions with data, and it captures the intuitive
notion that, in order to provide privacy to individuals, the results
of an interaction with a database should be almost the same whether
or not any particular individual is present in a database. This is a
strong notion of privacy that makes no assumptions about the power
or background knowledge of a potential adversary.
Overall, this work shows that modest revisions to a mobility model
drawn from real-world, large-scale location data allow for rigorous
demonstrations of its privacy without overly compromising its
utility. More broadly, it shows that there is reason for optimism
regarding the judicious use of Big Data repositories of potentially
sensitive information.
Mir presented preliminary results at the NetMob conference on mobile
phone datasets in May 2013. Versions of the work have since been
presented by Wright (as an example of differential privacy in use)
in a talk at the National Academy of Sciences Board on Research Data
and Information symposium in September 2013 and by Isaacman at the
IEEE International Conference on Big Data in October 2013.
DP-WHERE is part of Mir’s PhD dissertation, which she successfully
defended in August 2013, on the often conflicting goals of
extracting utility from data while preserving the privacy of
individuals. During her time at Rutgers, Mir was involved in a wide
range of DIMACS activities that include REU mentoring, co-authoring
a module for our Mathematics
for Planet Earth Project, and serving as a graduate mentor for
undergraduates participating in the Douglass-DIMACS Computing
Corps (DDCC).
The DDCC is featured in a recent article in the Daily
Targum and in a DIMACS News
highlight.
Printable version of this story: [PDF]
DIMACS Homepage
Contacting the
Center